The Rise of Growth Engineering: Future-Proofing Your Business in the Age of AI
Published: April 9, 2025
Looking back 15 years to my early days in growth marketing, two cultural artifacts define that era: the ubiquitous selfie stick 🤳🏻 and every marketer’s obsession with “growth hacking.” It was the era of SaaS startups booming, when clever tactics could make or break a company overnight. We were all searching for that magical combination of creativity and technical skill that would unlock exponential growth.
What fascinates me now isn’t how much has changed, but how much those early experiments paved the way for today’s more sophisticated approaches to finding growth. Back then, we were putting together growth strategies like amateur mechanics tuning a race car with duct tape and hope. Today, we’ve entered the era of precision engineering — where every decision is informed by data, every system is designed for scale, and growth becomes not just possible but predictable.
1. What Exactly is Growth Engineering?
Growth Engineering represents the evolution of marketing from art to science. It’s the disciplined application of engineering principles to business growth — combining software engineering’s rigor with marketing’s creativity and data science’s analytical power.
Growth Engineering is the disciplined application of engineering principles and its rigor, to business growth.
Imagine building a bridge. You wouldn’t rely on intuition to determine load capacity — you’d use physics, materials science, and precise calculations. Growth Engineering applies that same engineering mindset to customer acquisition, retention, and revenue expansion, aiming for predictable, scalable outcomes.
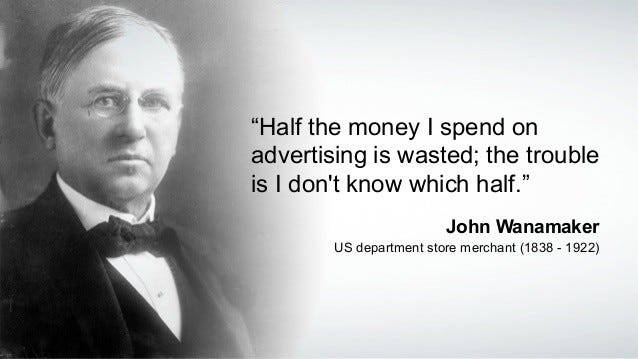
Modern implementations of this approach typically involve:
- a) Scientific Measurement Frameworks: 👉🏼 The days of guessing which 50% of your marketing budget is wasted are over. Today’s attribution models combine sophisticated techniques for a clearer picture:
- Multi-touch attribution (MTA) for granular channel impact analysis.
- Marketing mix modeling (MMM) for macro-level budget optimization.
- Incrementality testing (e.g., using Randomized Controlled Trials – RCTs, much like scientific clinical trials) to isolate the true, causal effect of campaigns.
- b) Predictive Infrastructure: 👉🏼 Beyond reporting, the real power comes from systems designed to anticipate and act:
- Automatically flagging anomalies in campaign performance or customer behavior.
- Recommending optimizations based on historical patterns and predictive models.
- Explaining hidden correlations between seemingly unrelated metrics to uncover new growth levers.
Source: Ladder.io blog
2. From “Hacks” to the Rigor of Engineering
The early days of growth hacking were exciting. Many teams achieved massive, short-term gains through clever automation and platform tricks. But like many shortcuts, their effectiveness often faded as platforms adapted and audiences grew savvier. These tactics lacked sustainability.
Growth Engineering solves this fragility by building robust systems that:
- Learn continuously: Every customer interaction — from website visits to support tickets — becomes training data, making the system smarter over time, unlike one-off hacks.
- Adapt automatically: When market conditions shift or platform algorithms change, the system can detect these changes and prompt adjustments or even reallocate resources within hours or days, not quarters.
- Scale predictably: Infrastructure designed with elasticity ensures that strategies effective for 1,000 customers can reliably scale to 100,000 or more.
While leading high-tech companies are heavily investing in fully automated, data-driven marketing engines, many businesses in other sectors are just beginning to realize the potential lying dormant in the vast amounts of data collected during their digital transformation journey.
For the most advanced companies pioneering Growth Engineering, sophisticated implementations include:
- Real-time bidding algorithms automatically optimizing ad spend second-by-second for maximum ROI.
- Dynamic creative engines personalizing not just messaging but entire user flows at scale, often fully automated.
- Closed-loop systems where sales outcomes automatically refine lead scoring models, improving funnel prediction accuracy.
- Self-balancing attribution models that continuously enhance reporting precision.
- A suite of Machine Learning (ML) models constantly fed by the Growth Engineering architecture, learning and producing ever more accurate business forecasts for leadership.
3. The Core Components of Growth Engineering
In my experience, a modern Growth Engineering capability rests on four interconnected pillars:
- a) Robust Data Infrastructure: Foundational to everything. Many companies discover their “data lake” is actually a swamp — full of unusable, inconsistent information. Building a solid foundation requires:
- Standardized Collection: Implementing consistent event tracking (like schema.org markup where applicable) across all platforms and touchpoints.
- Automated Cleaning Pipelines: Processes that identify, flag, and ideally correct data anomalies in near real-time.
- Unified & Accessible Storage: Utilizing modern data warehouses or platforms that allow relevant teams (including marketing) to access and query information efficiently, often without deep SQL expertise.
- b) A Culture of Experimentation: Rigorous testing is the engine of improvement. Leading teams run hundreds of controlled experiments annually, characterized by:
- Statistical Rigor: Using predefined calculations (like statistical power) to ensure results are valid and not just random noise.
- Systematic Deployment: Automated rollout of winning variations across relevant channels.
- Institutional Memory: Documenting results to avoid repeating failed tests and build collective knowledge.
- c) AI Orchestration: Moving beyond basic personalization to sophisticated automation and prediction:
- Predicting customer lifetime value (CLV) even during early interactions.
- Dynamically adjusting pricing or offers based on real-time signals (where applicable and ethical).
- Automatically generating and A/B testing thousands of creative variants (headlines, images, calls-to-action) to find optimal combinations.
- d) Organizational Alignment: Technology alone isn't enough; Growth Engineering thrives when silos break down:
- Shared Goals: Implementing shared OKRs (Objectives and Key Results) that unite engineering, marketing, product, and sales teams around growth outcomes.
- Collaborative Processes: Regular "growth reviews" where technical and creative teams jointly analyze results and brainstorm solutions.
- Aligned Incentives: Rewarding cross-functional collaboration and system-building, not just isolated campaign wins.
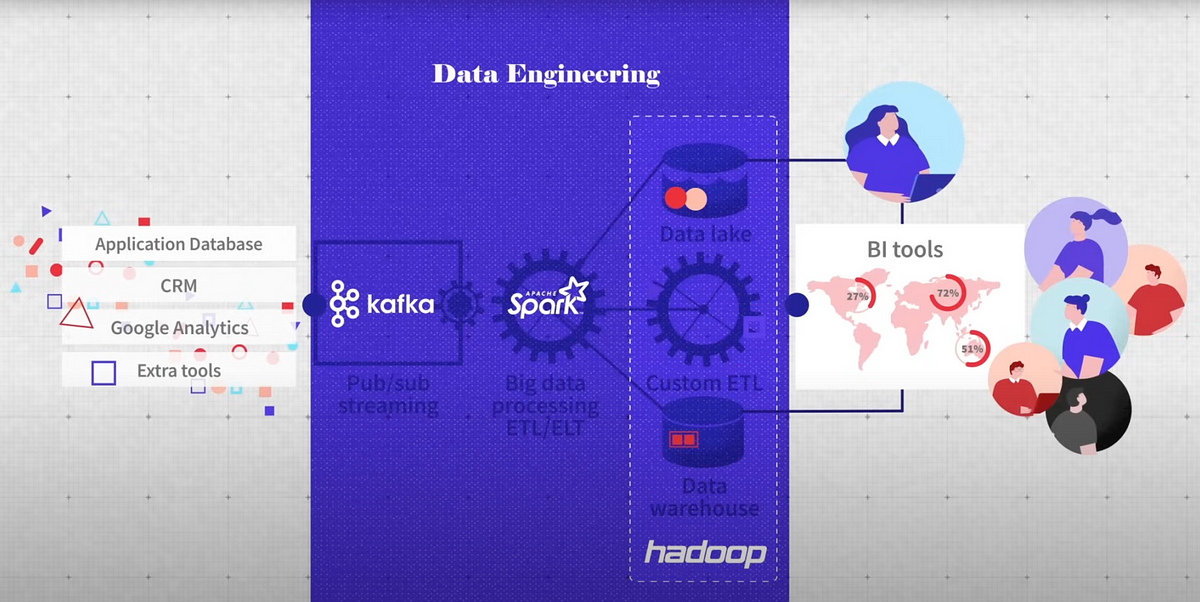
4. Why Growth Engineering Matters Urgently For Your Business
Due to rapid technological evolution, exponential data growth, and the rise of AI, three fundamental shifts make Growth Engineering essential for future-proofing your business:
- The Data Complexity Explosion: Customer journeys are increasingly fragmented, spanning thousands of potential touchpoints across multiple devices and channels. Without engineered systems, this leads to:
- Massive attribution gaps: Often, a significant portion (potentially 40%+) of touchpoints go unmeasured or misattributed.
- Wasted spend: Resources allocated to redundant or ineffective channels due to lack of clarity.
- Inability to identify true drivers: Difficulty discerning which activities genuinely influence conversion and long-term value.
- The Economic Imperative: In today's economy, demonstrating ROI is paramount. Growth teams must prove every dollar's impact through scientifically sound attribution. Furthermore:
- Efficiency is critical: Automating manual processes allows for leaner operations and faster response times.
- Competitive advantage: Identifying optimization opportunities and efficiencies before competitors becomes crucial for sustainable growth. Scalability means growing revenue cost-efficiently.
- The AI Revolution: AI tools are powerful, but their effectiveness hinges on the quality of the data foundation they are built upon. Growth Engineering provides:
- Clean data pipelines: Essential training fuel for accurate and reliable AI models.
- Standardized schemas: Enabling AI to learn from data across different departments and systems.
- Real-time data streams: Keeping predictive systems current and responsive.
Source: Monte Carlo Data blog
5. The Way Forward: Implementing Growth Engineering
For leadership teams ready to embrace this shift, here’s a potential roadmap, adaptable to your specific context:
Growth Engineering Implementation Timeline (Illustrative)
+---------------------------------------------------------------------------------------------------------+
| Months 1 - 3 | Months 4 - 6 | Months 7 - 12 | Months 12 - 18+ |
+==========================+==========================+=========================+=========================+> Time
| PHASE 1 | PHASE 2 | PHASE 3 | PHASE 4 |
| Laying the Foundation | Building Experimentation | Putting Data to Work | Scaling the System |
| | Muscle | | |
| - Data Audit | - Experimentation Culture| - Scale Data Processing | - Automate Processes |
| - Implement Standards | - Allocate Resources | - Enable Deeper Insights| - Expand AI Apps |
| - Build Data Literacy | - Establish Cadence | - Refine Measurement | - Institutionalize |
| | - First Predictive Models| - Enhance Visualization | - Democratize Insights |
+--------------------------+--------------------------+-------------------------+-------------------------+
| End of Month 3 | End of Month 6 | End of Month 12 | End of Month 18+ |
+---------------------------------------------------------------------------------------------------------+
- 🧱 Phase 1: Laying the Foundation (Months 1–3)
- Assess Current State: Conduct a thorough data audit, mapping all customer touchpoints and data sources. Identify gaps and inconsistencies.
- Implement Standards: Deploy robust tracking (e.g., server-side tagging via GTM, integrated CDP, CRM tracking) with consistent naming conventions.
- Build Data Literacy: Train key team members on data fundamentals, statistical thinking, and the importance of data quality.
- 👩🏽‍🔬 Phase 2: Building Experimentation Muscle (Months 4–6)
- Foster Culture: Create psychological safety for controlled testing within defined frameworks. Not every test will win, but every test provides learning.
- Allocate Resources: Dedicate a specific portion of time and budget (e.g., 10-15%) explicitly for experimentation.
- Establish Cadence: Implement an experimentation calendar and process for regular test cycles (e.g., weekly or bi-weekly sprints).
- Start Small & Focused: Develop initial predictive models (e.g., lead scoring) and dashboards connecting marketing spend to pipeline value.
- 📊 Phase 3: Putting Data to Work (Months 7–12)
- Scale Data Processing: Ensure capabilities exist to handle, enhance, and transform growing data volumes for various consumers (analysts, models, dashboards).
- Enable Deeper Insights: Utilize the infrastructure for proper Data Science exploration to answer strategic questions and identify trends.
- Refine Measurement: Build more accurate attribution models (potentially moving beyond basic MTA towards incrementality-focused MMM or RCTs).
- Enhance Visualization: Develop insightful dashboards tailored to different stakeholders (including leadership, potentially even in familiar tools like Excel if needed, but powered by robust backend data).
- 🚀 Phase 4: Scaling the System (Months 12–18+)
- Automate Core Processes: Implement automated bid management, budget allocation based on performance data, and dynamic creative optimization.
- Expand AI Applications: Explore AI use cases beyond marketing, such as in customer service (predictive support needs) or success (churn prediction).
- Institutionalize Knowledge: Develop playbooks, documentation, and internal training to embed Growth Engineering practices across the organization.
- Democratize Insights: Aim towards systems where management can ask key business questions directly via AI interfaces, leveraging the underlying data and models without needing constant data science intervention for standard queries.
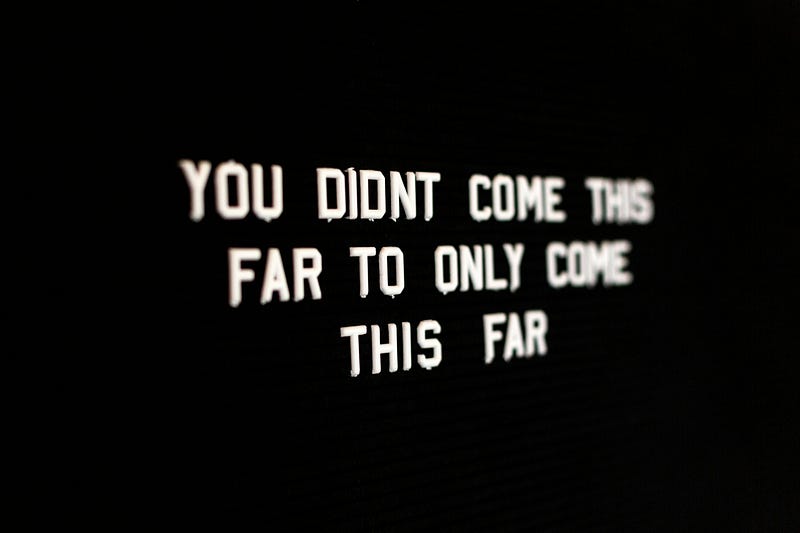
6. Conclusion: The New Growth Imperative
Growth Engineering is more than just adopting new tools; it means a fundamental shift in how businesses must approach growth to thrive in the data-driven, AI-powered future. The companies poised to lead in the coming decade will be those that:
- Treat growth as an engineering discipline demanding rigor, structure, and continuous improvement.
- Build intelligent systems that learn, adapt, and optimize automatically over time.
- Make critical decisions based on validated data and experimentation, rather than solely on hierarchy or intuition.
This transition requires strategic investment and commitment, particularly from leadership. However, the alternative—relying on outdated tactics in an increasingly complex and competitive environment—poses a far greater risk to long-term survival and success. Whether you are emerging from a digital transformation phase or seeking truly scalable, cost-efficient growth, the Growth Engineering paradigm—already embraced by tech leaders—offers a proven path forward.
The future belongs to those who engineer it. Will your organization be among them?
References
- Ladder’s Secret Weapon: Growth Engineering
- 9 Data-Driven Growth Strategies Small Businesses Can Implement Today
- Principles of Sustainable Engineering, PennState University
If you are a business leader grappling with growth and marketing challenges in this new era and want to discuss these concepts further, feel free to connect. You can find me on X (formerly Twitter) and LinkedIn.